2019 Blue Waters Symposium: Invited Speakers
David Keyes
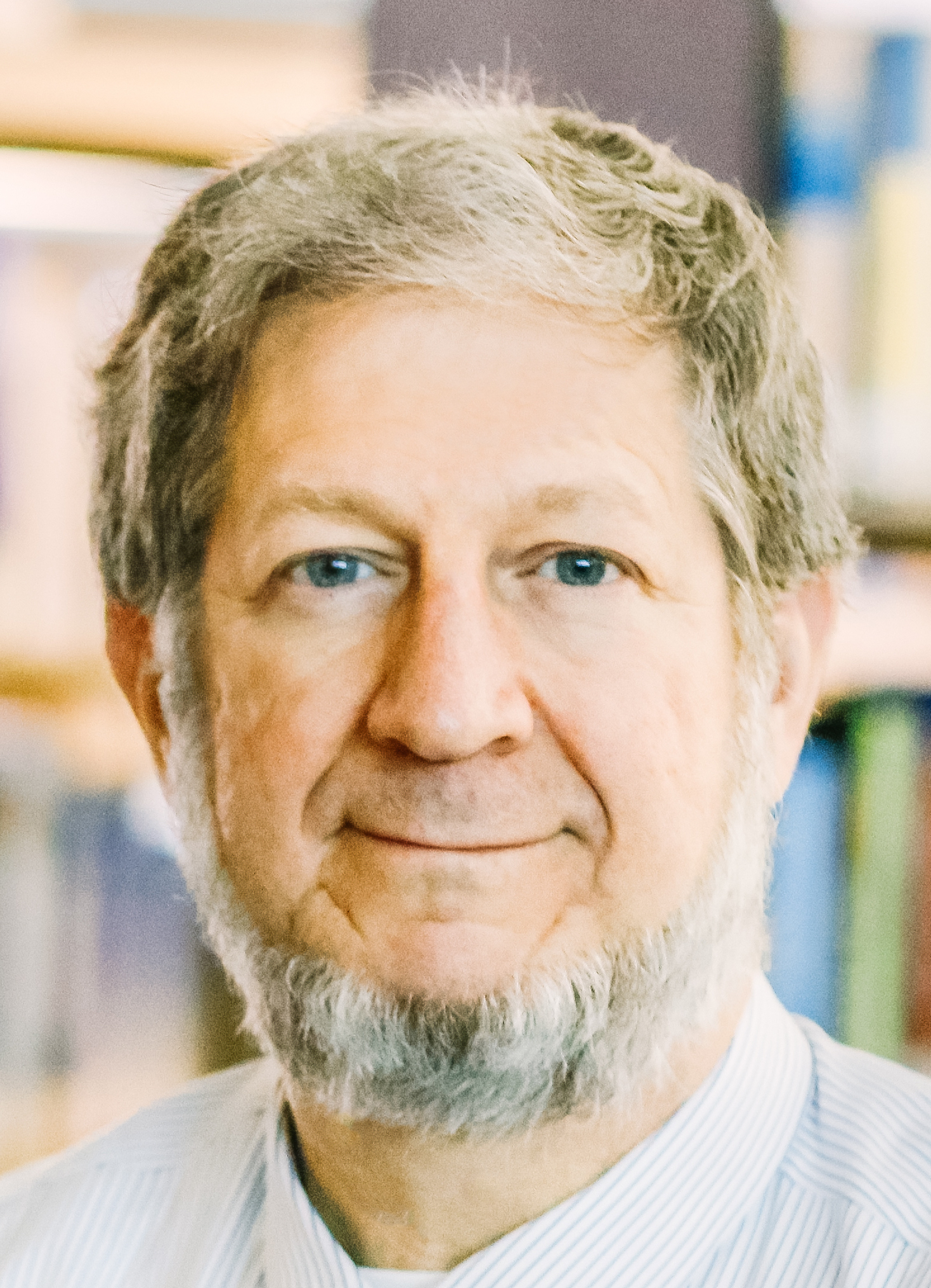
King Abdullah University of Science and Technology
David Keyes directs the Extreme Computing Research Center at the King Abdullah University of Science and Technology (KAUST), where he was the founding Dean of the Division of Mathematical and Computer Sciences and Engineering in 2009 and currently serves in the Office of the President as Senior Associate for international collaborations and institutional partnerships. He works at the interface between parallel computing and the numerical analysis of PDEs, with a focus on scalable implicit solvers. Newton-Krylov-Schwarz (NKS) and Additive Schwarz Preconditioned Inexact Newton (ASPIN) are methods he helped name and is helping to popularize. Before joining KAUST as a founding dean in 2009, he led multi-institutional scalable solver software projects in the SciDAC and ASCI programs of the US DOE, ran university collaboration programs at LLNL's ISCR and NASA's ICASE, and taught at Columbia, Old Dominion, and Yale Universities. He is a Fellow of SIAM, AMS, and AAAS, and has been awarded the ACM Gordon Bell Prize, the IEEE Sidney Fernbach Award, and the SIAM Prize for Distinguished Service to the Profession. He earned a BSE in Aerospace and Mechanical Sciences from Princeton in 1978 and a PhD in Applied Mathematics from Harvard in 1984.
The Convergence of Big Data and Large-scale Simulation
Motivations abound for the convergence of large-scale simulation and big data: (1) scientific and engineering advances, (2) computational and data storage efficiency, (3) economy of data center operations, and (4) the development of a competitive workforce. To take advantage of advances in analytics and learning, large-scale simulations should incorporate these technologies in-situ, rather than as forms of post-processing. This potentially reduces IO, may obviate significant computation in unfruitful regions of physical parameter space, offers smart data compression, and potentially improves the results of the simulation, itself, since many simulations incorporate empirical relationships currently tuned by human experts. Flipping the perspective, simulation potentially provides significant benefits to analytics and learning workflows. Theory-guided data science is an emerging paradigm that aims to improve the effectiveness of data science models, as a form of regularization, wherein non-unique candidates are penalized by physical constraint. Simulation can also provide training data for machine learning. Finally, much software has been developed for large-scale simulation, particularly in data-sparse linear algebra and second-order optimization, that promises to expand the practical reach of analytics; while the complex parameterized codes of large-scale simulation may in turn be tuned for computational performance by machine learning - applying machine learning to the machine.
Rick Stevens
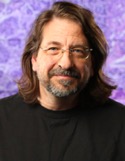
Argonne National Laboratory and University of Chicago
Professor Rick Stevens is internationally known for work in high-performance computing, collaboration and visualization technology, and for building computational tools and web infrastructures to support large-scale genome and metagenome analysis for basic science and infectious disease research. He is the Associate Laboratory Director at Argonne National Laboratory, and a Professor of Computer Science at the University of Chicago. Currently, he is the principle investigator of the NIH-NIAID funded PATRIC Bioinformatics Resource Center, the Exascale Computing Project (ECP) Exascale Deep Learning and Simulation Enabled Precision Medicine for Cancer project, and the predicitive models pilot of the DOE-NCI funded Joint Design of Advanced Computing Solutions for Cancer (JDACS4C) project. Over the past twenty years, he and his colleagues have developed the SEED, RAST, MG-RAST, and ModelSEED genome analysis and bacterial modeling servers that have been used by tens of thousands of users to annotate and analyze more than 250,000 microbial genomes and metagenomic samples.
AI for Science
In this talk, I will describe an emerging initiative at Argonne National Laboratory to advance the concept of Artificial Intelligence (AI) aimed at addressing challenge problems in science. We call this initiative AI for Science. The basic concept is threefold: (1) to identify those scientific problems where existing AI and machine learning methods can have an immediate impact (and organize teams and efforts to realize that impact); (2) identify areas of where new AI methods are needed to meet the unique needs of science research (frame the problems, develop test cases, and outline work needed to make progress); and (3) to develop the means to automate scientific experiments, observations, and data generation to accelerate the overall scientific enterprise. Science offers plenty of hard problems to motivate and drive AI research, from complex multimodal data analysis, to integration of symbolic and data intensive methods, to coupling large-scale simulation and machine learning to drive improved training to control and accelerate simulations. A major sub-theme is the idea of working toward the automation of scientific discovery through integration of machine learning (active learning and reinforcement learning) with simulation and automated high-throughput experimental laboratories. I will provide some examples of projects underway and lay out a set of long-term driver problems.
Dan Stanzione
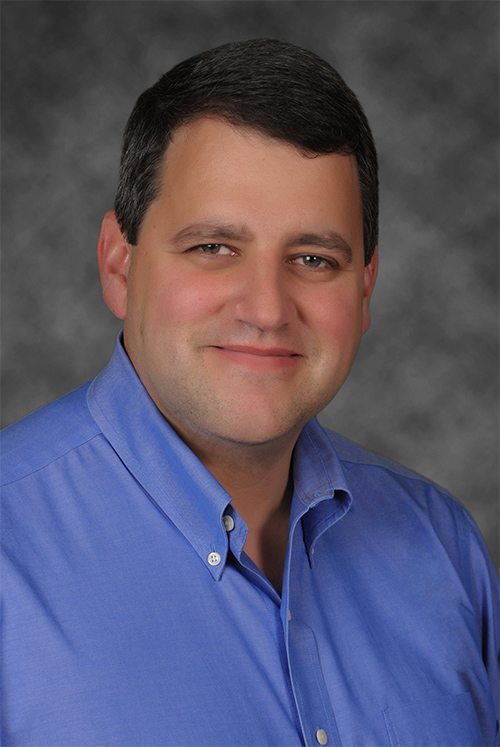
Texas Advanced Computing Center (TACC) and University of Texas at Austin
Dr. Dan Stanzione, Associate Vice President for Research at The University of Texas at Austin since 2018 and Executive Director of the Texas Advanced Computing Center (TACC) since 2014, is a nationally recognized leader in high performance computing. He is the principal investigator (PI) for several projects including a multimillion-dollar National Science Foundation (NSF) grant to acquire and deploy Frontera, which will be the fastest supercomputer at a U.S. university. Stanzione is also the PI of TACC's Stampede2 and Wrangler systems, supercomputers for high performance computing and for data-focused applications, respectively. He served for six years as the co-director of CyVerse, a large-scale NSF life sciences cyberinfrastructure in which TACC is a major partner. In addition, Stanzione was a co-principal investigator for TACC's Ranger and Lonestar supercomputers, large-scale NSF systems previously deployed at UT Austin. Stanzione received his bachelor's degree in electrical engineering and his master's degree and doctorate in computer engineering from Clemson University, where he later directed the supercomputing laboratory and served as an assistant research professor of electrical and computer engineering.
Frontera: The Next NSF Leadership Computing Resource
In this talk I will describe the new NSF-funded Frontera system, including the hardware specifications, software capabilities, and an overview of the entire project. I will also provide new updates on the system deployment, benchmarking to date, and (very) early science results. A discussion will follow of how users can get access to the new system, as well as contribute to planning for new system capabilities and support activities, as well as to requirements for the design of future NSF computing resources.
Brian Bates and Cathleen Williamson: National Geospatial-Intelligence Agency
The National Geospatial-Intelligence Agency and its Work
The National Geospatial Intelligence Agency is both a combat support agency under the United States Department of Defense and an intelligence agency of the United States Intelligence Community, with the primary mission of collecting, analyzing, and distributing geospatial intelligence (GEOINT) in support of national security. In addition to using GEOINT for U.S. military and intelligence efforts, the NGA provides assistance during natural and man-made disasters, and security planning for major events such as the Olympic Games and Hurricane Katrina. NGA also engages with research and innovation to improve being able to address their missions, including a vision to evolve from maps to models of the world.